Is there a trade-off between privacy & discrimination in algorithmic decision making?
The Issue
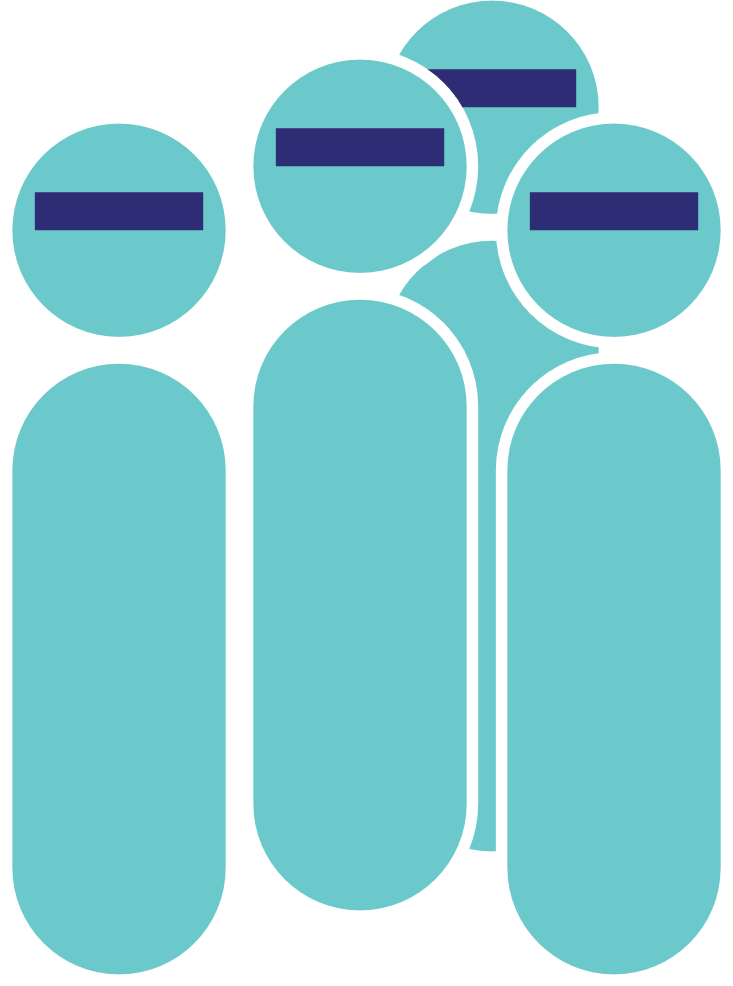
He categorised bias across three dimensions:
1. Human bias: There can be bias among the people who create the algorithm that gets translated into the data-processing mechanism
2. Sample bias: there can be bias in the data sample that is used by the algorithm
3. Societal bias: there can be data that has inequality embedded in a way that leads to discriminatory outcomes.
Indirect discrimination occurs when there is a policy or rule that appears to be neutral and the same for everyone but in fact has the effect of disadvantaging someone with a particular attribute. A useful example comes from the insurance industry4. According to the Institute of Actuaries Australia, engine size of a vehicle is found to be a good predictor of car insurance claim costs. The causal relationship being that the larger the engine the more powerful the car, the more damage it can do when it hits something. It is also generally accepted that engine size is correlated with gender. Males tend to drive cars with bigger engines. This means that if engine size is used in rating car insurance premiums, it might be said to cause indirect discrimination against men.
Many datasets are likely to include data which is correlated, at least to some degree, with a protected attribute yet the actual protected attribute is not within the dataset itself. Since there are a large number of protected attributes included under legislation, this means that the removal of protected attributes from a dataset is no protection from discrimination. As The Gradient Institute reveals5, (a partner of Ethical AI Advisory) omitting the protected attribute from the data set can actually lead to worse outcomes in some scenarios.
So how can organisations navigate through these tricky sometimes conflicting legal requirements of protecting people’s privacy on the one hand yet having enough information to assess both direct and indirect discrimination on the other?

Our View
For governments and policy makers there is a real opportunity for regulators to work together in developing regulatory sandboxes which can enable organisations to confidently experiment with sensitive information in a safe environment to detect and mitigate biases or privacy issues. In this current environment where AI technologies and their applications are racing ahead of existing legal frameworks, regulators need to be working closely with industry to support ethical innovation, rather than only responding when legal breaches occur.
[1] In the Australian Capital Territory the following attributes are: disability • sex • race • sexuality • age • gender identity • relationship status • status as a parent or carer • pregnancy • breastfeeding • religious or political conviction • guide dog or other assistance animal • industrial activity • profession, trade, occupation or calling • spent criminal conviction • association with a person who has an attribute listed above.
[2] https://humanrights.gov.au/our-work/employers/quick-guide-australian-discrimination-laws
[3] Ignacio N. Cofone, Algorithmic Discrimination Is an Information Problem, 70 Hastings L.J. 1389 (2019).
Available at: https://repository.uchastings.edu/hastings_law_journal/vol70/iss6/1
[4] https://actuaries.asn.au/Library/Miscellaneous/2020/ADWGPaperFinal.pdf
[5] https://gradientinstitute.org/blog/2
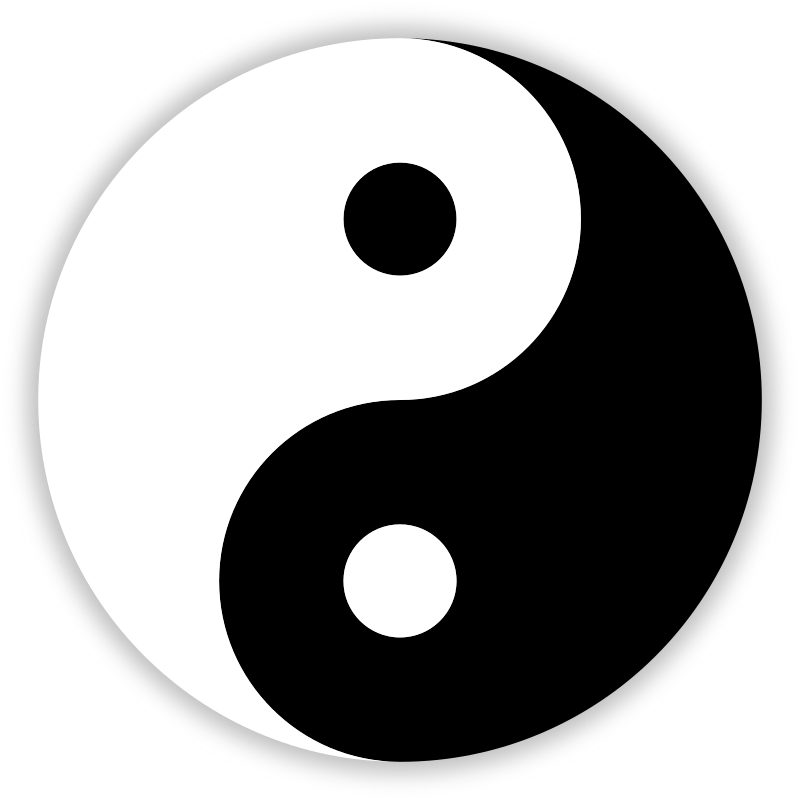