What is ‘High Risk’ AI?
The Issue
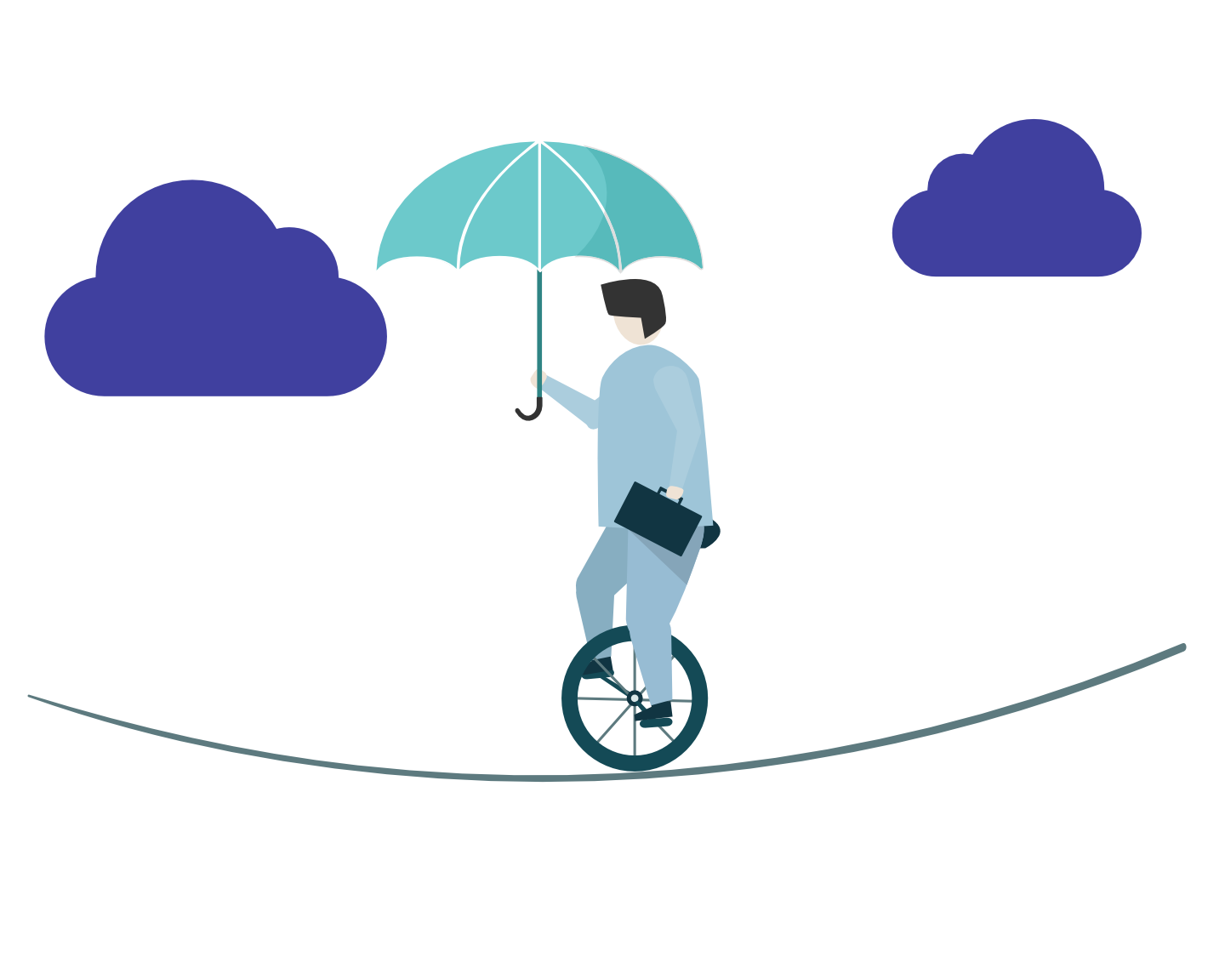
This would therefore not be ‘high risk AI’?
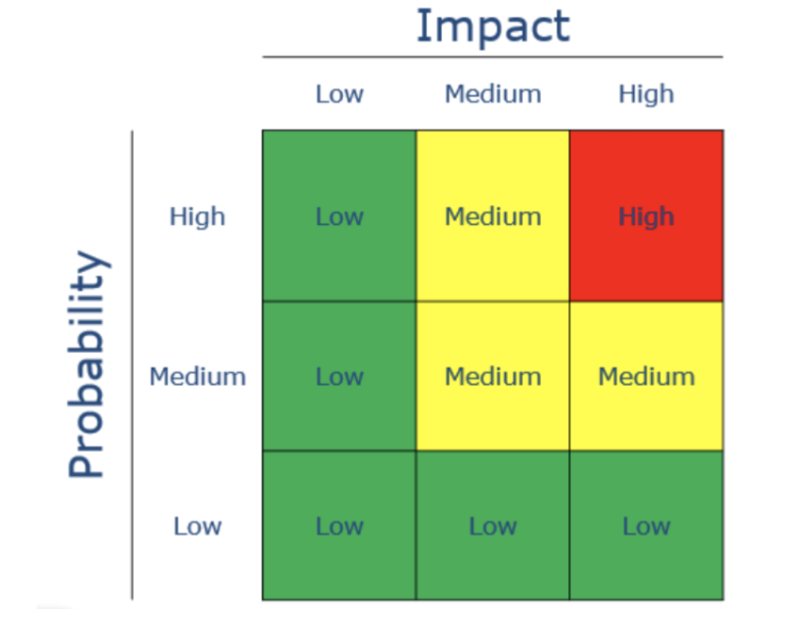
The European Commission have added another dimension to their risk framework by identifying ‘high risk sectors’. So an AI application should be considered high-risk if it involves both a high-risk sector (healthcare, transport, energy and parts of the public sector) and high-risk use. Only if these two conditions are met, would it fall under specific regulations and governance structures[1]. So under this general criteria the assumption is that AI’s impact will never be high risk outside of these industries?
Intensity of potential harm (x-axis)
Impact on fundamental rights, equality or social justice
Number of people affected
Impact on society as whole
Dependence on the decision (y-axis)
Control: Decisions and actions of an AI system filtered through meaningful human interaction imply a lower threshold for ethical review than machines acting without human intermediaries.
Switchability: the ability to change the AI system for another or avoid being exposed to an algorithm decision by opting out of using specific services.
Redress: challenging or correcting and algorithmically made decision and the time needed to follow up on a request adequately. Machine-made decisions that cannot be challenged at all increase the dependence on the decision.
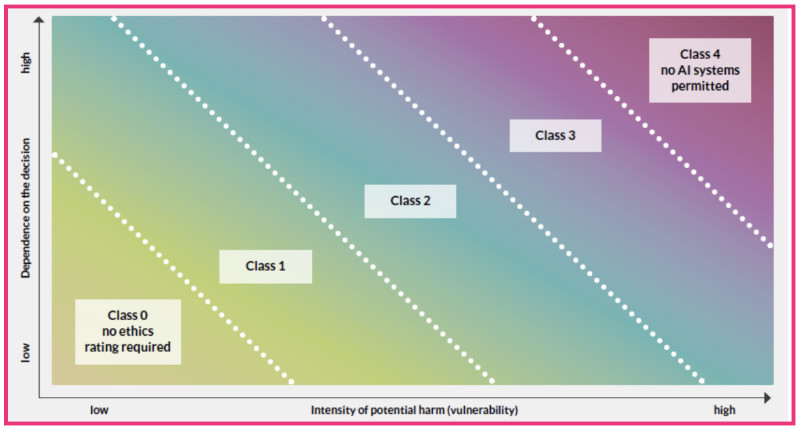
Source: From Principles to Practice: An interdisciplinary framework to operationalise AI ethics
Krafft, Tobias & Hauer, Marc et al. (2020). https://www.researchgate.net/publication/340378463_From_Principles_to_Practice_-_An_interdisciplinary_framework_to_operationalise_AI_ethics
Our View
Tools such as these, and others are useful and necessary when developing and deploying automated decision systems and AI. However, their value will be limited unless a multidisciplinary team or a cross section of potentially affected stakeholders are also invited to participate in the risk assessment process. Additionally, the concept of AI ‘impact’ or ‘harm’ needs to be unpacked as this concept can mean different things depending on the ethical, legal, corporate or social lens that is applied. We will explore this in a following blog.
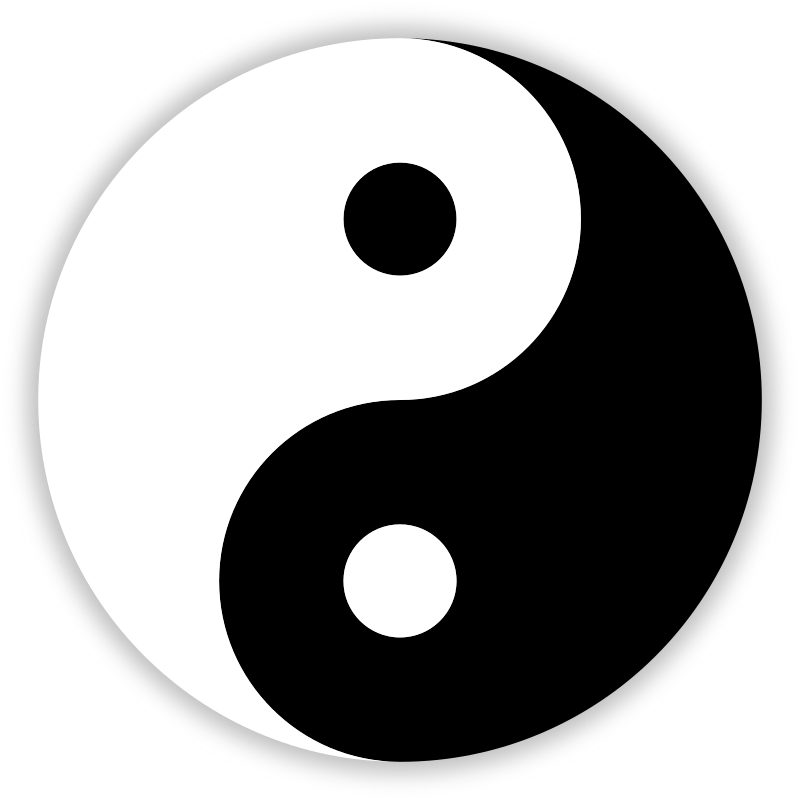