Bad Robots – Policing Algorithms Reinforce Existing Racial & Socioeconomic Biases
Bad Robot Outcome:
Police departments have turned to Artificial Intelligence as a way to increase efficiency and more precisely target crime. However, what happens when the algorithmic tools they elect to use end up reinforcing, or even worsening, longstanding and problematic biases toward BIPOC and the communities in which such individuals live?
The Story
Dozens of police departments throughout the United States use location-based algorithms to help them make predictions as to when and where crime will happen within their jurisdictions. This technology creates links between places, events, and historical crime data in an attempt to anticipate future occurrences (for example, whether crime is more likely to occur at certain times of day, or at large gatherings such as concerts, etc.).One such location-based tool is called PredPol and is used by dozens of cities throughout the United States. This technology uses machine learning to make its predictions. More specifically, about two-to-five years of three data points (crime type, crime location, and crime date/time location) taken from police departments’ records are ingested by the algorithm. The company’s website makes a point to note that no personally identifiable information is used in the process, so as to eliminate “the possibility for privacy or civil rights violations.”
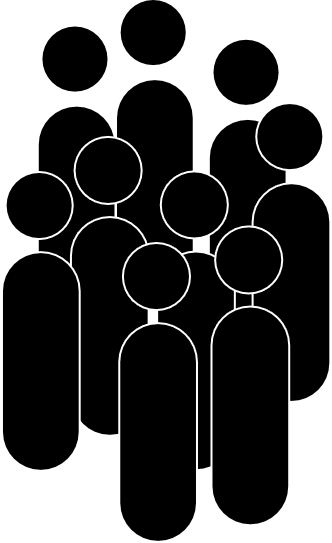
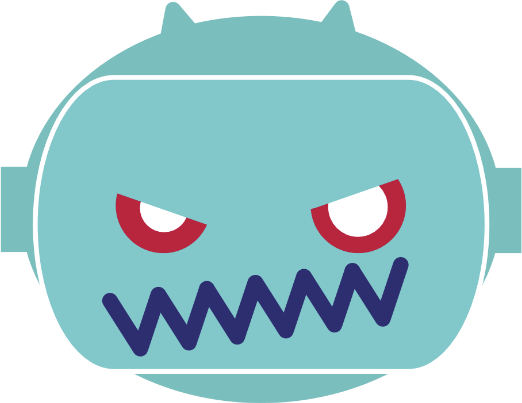
PredPol then uses the data points listed above to divide cities into 500-by-500 foot blocks that are color-coded according to the predictions made by the algorithm. Blocks designed as red are considered “high risk” and police officers are encouraged to spend at least 10% of their time there. The algorithm is updated daily.
The accuracy and effectiveness of the technology, used by over 50 police departments in the United States, as well as a handful in the UK, is still hotly contested.
The Fall-Out
Some PredPol users have noted positive results. For example, in the English city of Kent street violence fell by 6% following a four month trial of the software. Steve Clark, deputy chief of the Santa Cruz, California police department, heralded the technology as being “incredibly accurate at predicting the times and locations where these crimes were likely to occur.”However, concerns and criticisms continue to pile up. In June over 1,400 mathematicians from around the United States signed a letter urging their colleagues to stop collaborating with the country’s police agencies, due to their disparate treatment of people of color. In particular the letter focused on predictive policing technologies, like PredPol.
Their letter argues that such algorithms are inherently biased because they are seeded with data from past arrests, which have long been documented to be fraught with racial biases themselves. For example, in the United States, a Black person is five times more likely than a white person to be stopped by a police officer without cause.
As noted by Franklin Zimring, a criminologist at the University of California, Berekely, “if a police presence itself is a biasing influence on measurable offense volume, you shouldn’t use those events as a basis for allocating police resources, or it’s a self-fulfilling prophecy.”
Despite both the positive feedback from deputy chief Clark, as well as the fact that PredPol is actually headquartered in the city, Santa Cruz became the first city in the US to ban predictive policing technology. As stated by their first Black male mayor, “we have technology that could target people of color in our community – it’s technology that we don’t need.” Furthermore, the city’s police chief noted that PredPol ceased to be used after his first six months on the job and there was no significant corresponding change in the crime rate.

Our View
The use of predictive policing algorithms cuts to the core of two of the eight AI Ethics Guidelines that we at the Ethical AI Advisory have adopted ourselves and that we encourage both private and public entities to also adopt. The first is fairness. The use of Artificial Intelligence should not produce unjust results against certain communities or individuals. Unfortunately, predictive policing does just that. Because crime data is skewed by underlying racial and socioeconomic biases, its use within policing algorithms produces results that exacerbate and amplify such biases. Therefore, even though PredPol does not utilize personal information and/or racial/soci-economic data within its algorithm, the results are still unfair due to the inherently biased crime data that is used. The second is transparency. To be fair, PredPol is rather transparent with how their algorithm works. You can read about it on their website. However, the lack of transparency here sits with cities and police departments, perhaps even less so than with tools like PredPol. As noted by Rashida Richardson, director of policy research at the AI Now Institute, “we don’t know how many police departments have used, or are using, predictive policing.” Given that this technology impacts both the allocation of public resources, as well as which citizens are more targeted by police efforts, absolute transparency is essential.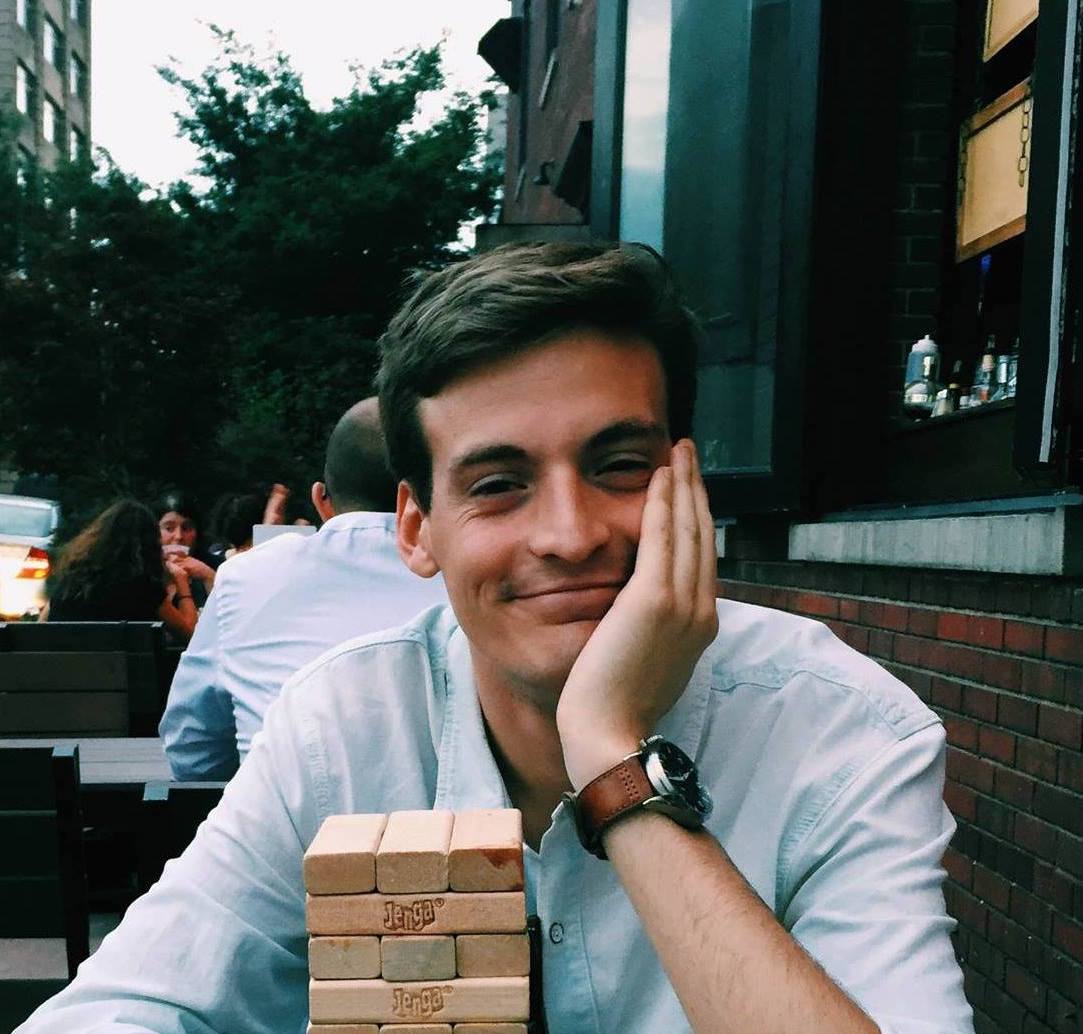
Written by: