Open Loop – Facebook’s policy prototyping sandbox.
Open Loop is a collaborative initiative supported by Facebook to contribute practical insights into policy debates by prototyping and testing approaches to regulation before they are enacted. Putting aside one’s natural apprehension about the motivation for Facebook’s involvement in such an exercise, Open Loop and initiatives like it are good and very welcome.
The calls for regulation of AI have been strong, particularly in Europe, for some time, but regulation can be a very blunt instrument and those crafting legislation are not always best placed to understand the practicalities of a business needing to adhere to particular laws. It can also be difficult to anticipate the effects produced by laws before they are enacted and in an emerging technology such as AI which is ill defined and includes a complex ecosystem of actors, the challenge of creating practical and fit for purpose AI regulation is great. This is where policy prototyping comes in.
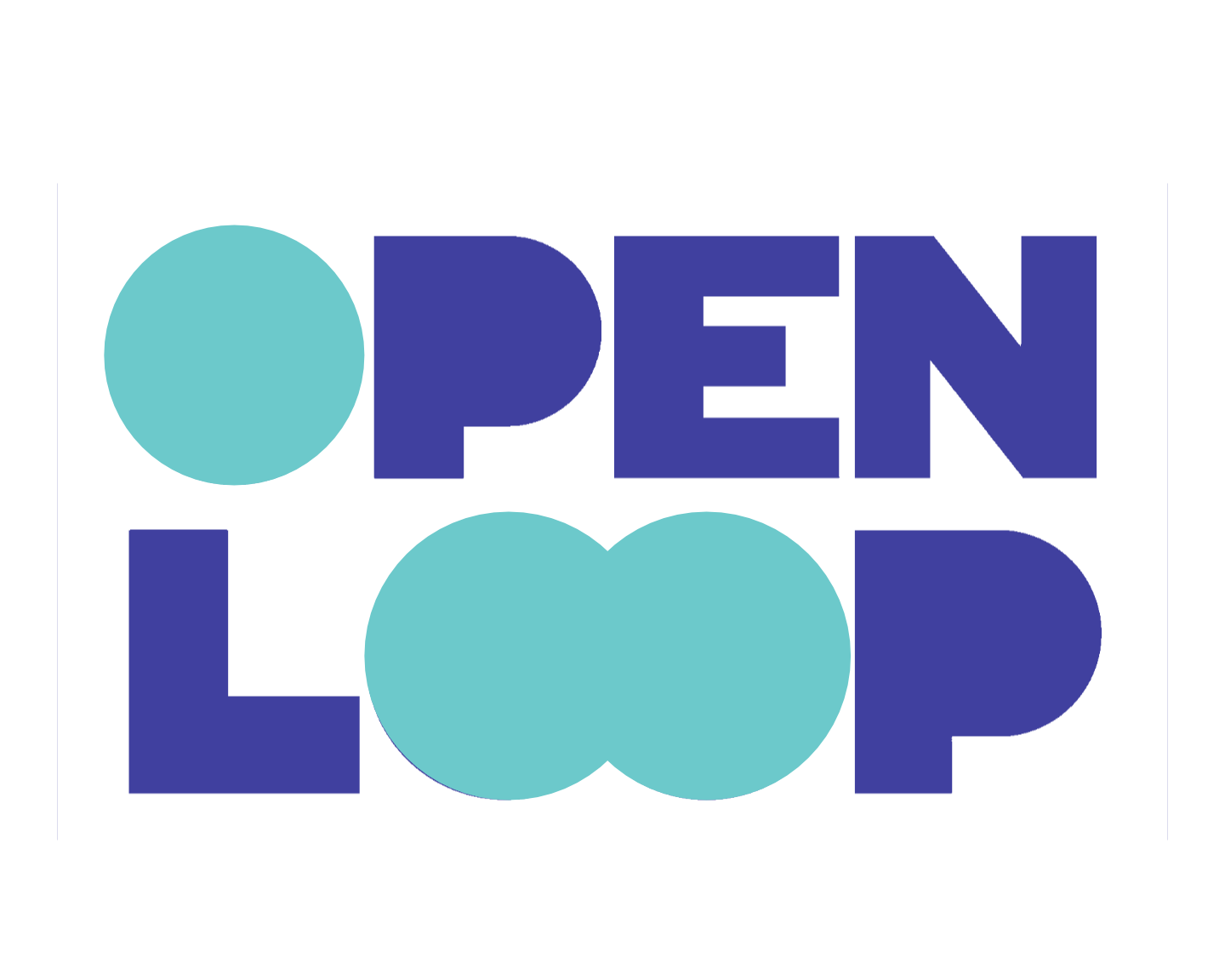
Policy prototyping is a methodology to test the efficacy of a policy by first implementing it in a controlled environment. Regulatory sandboxes have been around for a little while, particularly in the FinTech space, but have only recently emerged in relation to AI.
In its European project, Open Loop partnered with 10 European AI companies to co-create an Automated Decision Impact Assessment ADIA framework (policy prototype) that those companies could test by applying it to their own AI applications.
The policy prototype was structured into two parts:
The researchers assessed the policy prototyping across three dimensions: policy understanding, policy effectiveness and policy cost. A detailed description of the experiment, findings and recommendations is available in a report here.
Our View
A key finding of the study was that a procedural approach to assessing AI risk was more practical for the companies than codified prescriptions of what is high or low risk AI. This makes intuitive sense but it does place a significant onus on companies to establish internal governance procedures, have a thorough understanding of the range of potential AI harms and risk thresholds related to the AI service they offer and have the capability to mitigate those risks appropriately. This, of course, is the great value of policy prototyping or regulatory sandboxes, in the sense they give organisations an opportunity to safely test their applications against a set of criteria to see how they measure up.
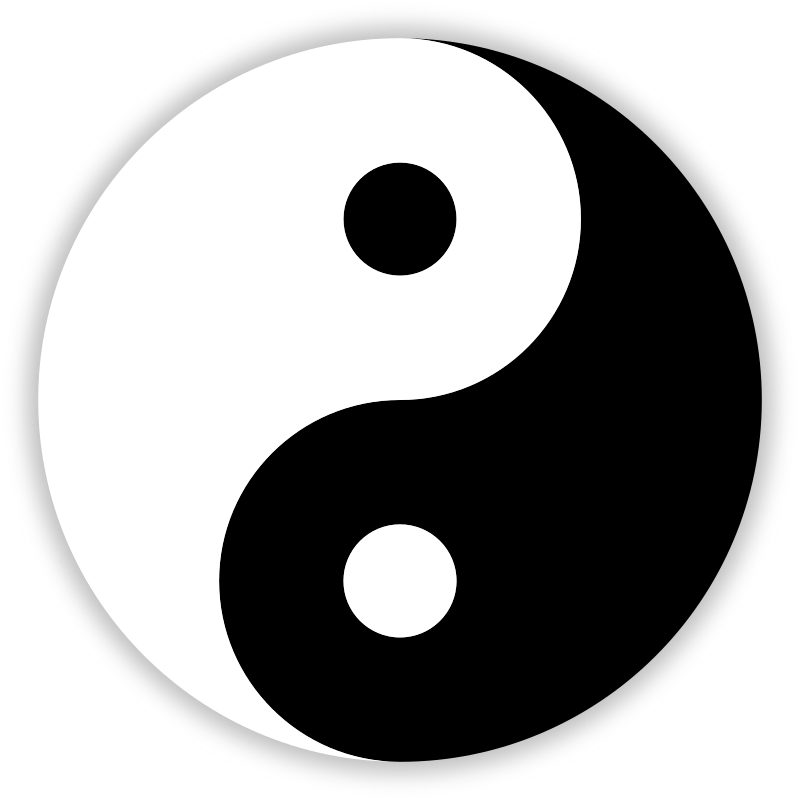