Can AI be used to promote equality in healthcare access?
The Issue
Access to healthcare remains a pressing concern globally, especially among minority communities. The inherent societal bias has been a significant concern across the healthcare spectrum, especially in the wake of growing economic and social disparity.
With the increasing adoption of AI technologies in different areas, there has been rising hope that its adoption in healthcare will help improve on existing medical technology, personalised medicine and provide reprieve and equity of access to the underserved and socially disadvantaged communities.
However, the adoption of AI in healthcare must overcome critical challenges to ensure that AI technologies do not amplify the existing biases, which would render such a promoter of healthcare access inequality.
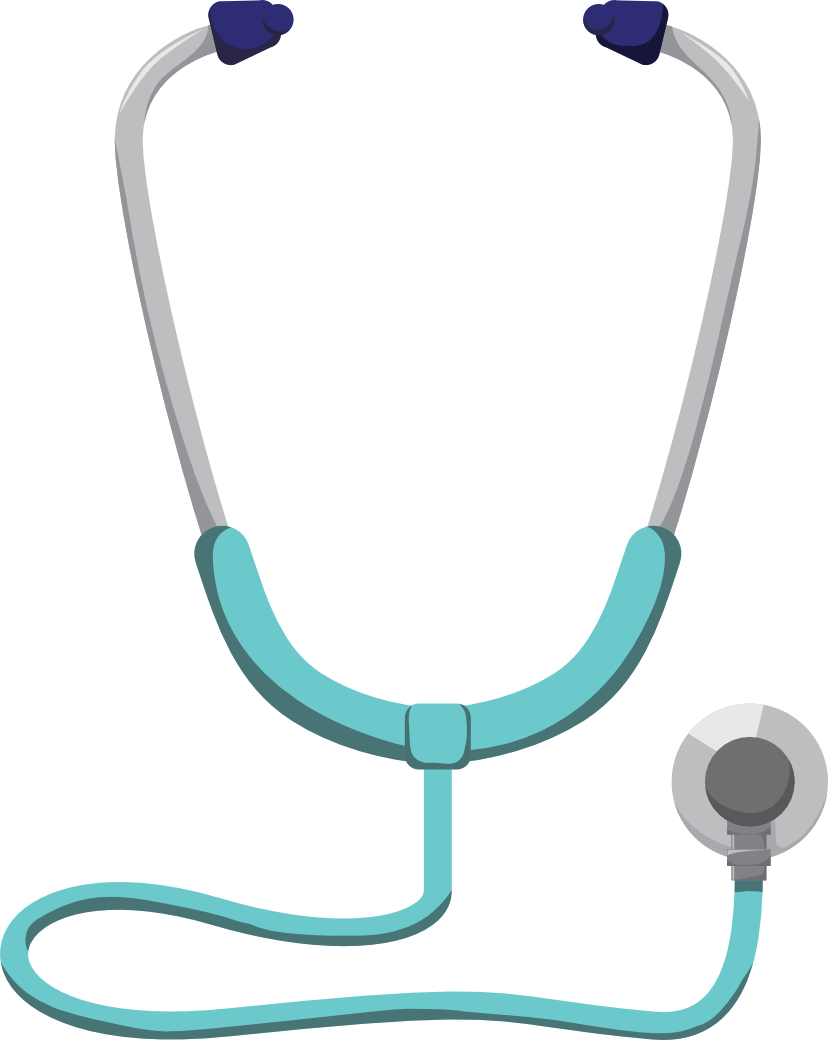
AI and equality in access
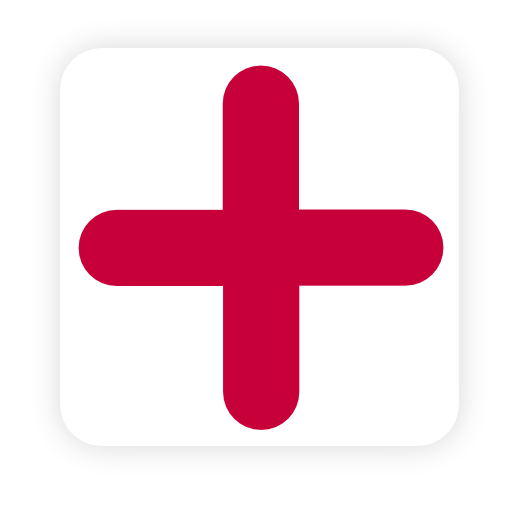
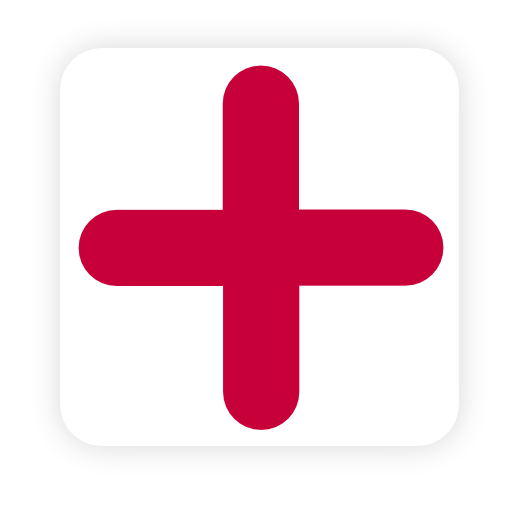
Our View
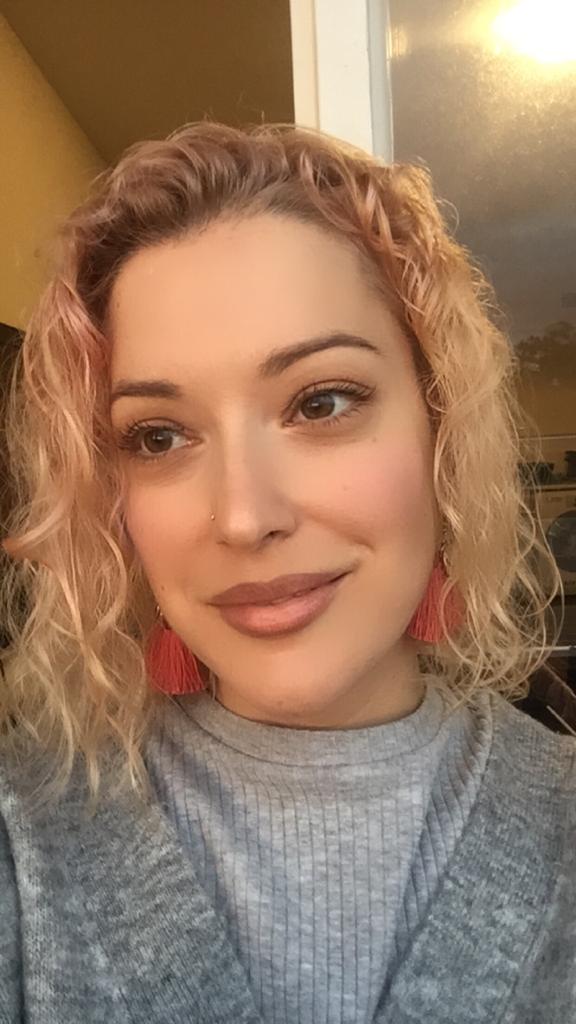
Written by: